Implementation and Learning
Once a decision is made, the process enters the implementation phase. In some cases, particularly for lower-level decisions, planners will have direct control over the implementation process. This is an ideal scenario, in that there is high assurance that the plan will be implemented as intended.
More commonly, decisions take the form of rules or plans that are carried out by others. This is a major reason for including stakeholders in the decision process. Stakeholders can provide insight into implementation concerns, including feasibility, cost, and likelihood of success. This improves decision making and also helps to ensure that the alternatives can be implemented as specified.
Biodiversity Monitoring
For some SDM applications, a decision marks the end of the process. This is uncommon with conservation applications because threats to biodiversity are rarely fully resolved. Plans are developed, then periodically revised in response to new knowledge, changing conditions, and changing objectives. Thus, the implementation phase of one plan is also the preparation phase of the next plan (Fig. 10.1).
Learning is a critical component of the preparatory phase, and it occurs mainly through monitoring and research. We will begin our discussion of this topic with an examination of generic biodiversity monitoring programs. Then we will consider monitoring and research programs that are developed and implemented as part of individual SDM processes.
Generic biodiversity monitoring programs are concerned with tracking the overall status of biodiversity as well as threats to biodiversity (CCRM 2010). They are intended to reveal management successes as well as problems that require alternative approaches or additional effort. They are also intended to identify new and emerging concerns and bring these to the attention of managers and the public. Finally, the measurement of biodiversity within natural areas is used to establish ecological baselines and to characterize the natural range of variation.
The state of biodiversity at a given point in time is an integrative measure affected by natural and anthropogenic disturbances and by decisions taken at all levels of the decision hierarchy. The main indicators of biodiversity status at the species level are abundance and distribution over time. At the ecosystem scale, the focus is on ecological integrity, measured in terms of composition, structure, and function at multiple scales.
Biodiversity monitoring programs also track potential threats, especially industrial processes that cause disturbance or release waste into the environment. Tracking progressive changes in the overall anthropogenic footprint (i.e., cumulative effects) is of particular interest because this type of information is difficult to assemble from project-level monitoring. By combining long-term datasets on human activities with biodiversity trends, causal relationships that are critical for conservation decision making can be identified.
In practice, our ability to monitor biodiversity is severely constrained by funding limitations, institutional factors, and practical feasibility. Responsibility for wildlife management is fragmented, so there is no comprehensive, national-scale biodiversity monitoring program in Canada. The federal government oversees the monitoring of a few select species groups, such as migratory birds. It also conducts satellite-based monitoring of select biophysical attributes (Wulder 2011). Citizen-science programs, such as the Breeding Bird Survey, also contribute to national-scale assessments (Sauer and Link 2011). As for species at risk, status assessments are coordinated nationally, but the information used to make the assessments comes from diverse local sources (CESCC 2022).
At the provincial/territorial scale, biodiversity monitoring is highly variable. Monitoring programs are conducted at different spatial and temporal scales, measure different parameters, and use different protocols for data collection and analysis (CCRM 2010). The result is an information patchwork with many inconsistencies and gaps.
Focal species, including species at risk and species that are harvested, generally receive the most attention. Many of these species are regularly surveyed, providing ongoing estimates of abundance and distribution. The level of effort varies from province to province, depending on budget priorities and the perceived importance of a given species. Many species at risk are rare and/or difficult to census. Therefore, status assessments are often based on expert opinion rather than systematic surveys. The monitoring of non-focal species and ecosystem integrity usually receives a much lower priority, and is rudimentary in many parts of the country, especially in the north. Alberta’s comprehensive, province-wide system of biodiversity monitoring merits special mention because it provides an example of what can be accomplished (Box 10.6). Unfortunately, in the 20 years since its conception, Alberta’s monitoring program has not been emulated by other provinces.
The information collected through broad biodiversity monitoring programs is meant to inform the policy development process and provide context for lower-level decisions. However, this feedback loop is generally unstructured, partly because the policy development process is itself mostly unstructured, and partly because the information is scattered and difficult to assemble. In many cases, poor institutional linkages also hinder the process. For example, considerable effort is expended in monitoring ecological integrity within national parks; however, the linkages needed to apply this valuable baseline information on the working landscape are often lacking.
Box 10.6. The Alberta Biodiversity Monitoring Institute (ABMI)
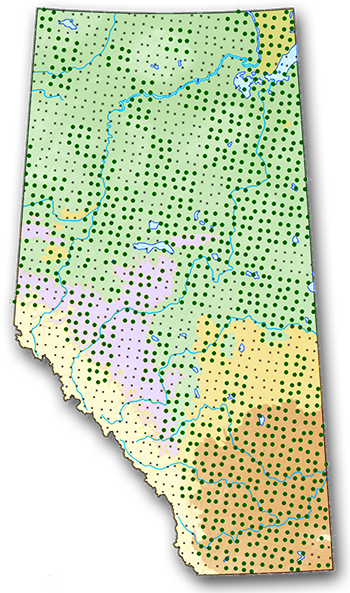
The ABMI monitoring program uses a grid of 1,656 sites distributed evenly across Alberta at a 20 km spacing (Fig. 10.9). Currently, 150 sites are surveyed annually based on a budget of $13 million, which includes data analysis and reporting (ABMI 2017a). Terrestrial samples are taken across a 1 ha plot. Birds are individually counted at nine point-count locations, mammal presence is recorded along linear transects, and the presence of vascular plants is recorded in four sample sites. Aquatic samples are taken from the nearest wetland. Bryophyte, lichen, and mite specimens are collected in the field and later identified in a laboratory. Site conditions are also recorded during the site visit.
In recent years, automated cameras and acoustic recorders have been added to the sampling protocol (ABMI 2017b). Efforts are also underway to monitor rare animals and plants, which require specialized sampling methods.
In addition to the site sampling, AMBI uses remote sensing to document the human footprint, including disturbances related to agriculture, forestry, oil and gas, transportation, and human habitation. Fine-scale data (1:5,000) are collected annually within 3×7 km rectangles centred on each survey site, and coarse-scale data (1:15,000) are collected every two years for the entire province. ABMI also maintains a provincial-scale GIS database that includes detailed information on vegetation classes, soils, climate, and other landscape attributes.
An integrated science centre uses the collected data to develop models that relate species abundance to habitat information and human disturbance. With these models, province-wide interpolated maps of species distribution and abundance have been created for over 800 species. Other mapping products predict the species-level changes that have occurred as a result of development. Species and habitat data, mapping products, and analytical reports are all publicly available at no charge through an integrated web-based data portal (www.abmi.ca).
Outcome Monitoring
Monitoring is also conducted to support individual management plans. Indeed, under SDM, the acquisition of knowledge between decision cycles is a formal part of the decision process (Runge 2011). Using a structured approach for learning ensures that monitoring efforts are efficient and tailored to the specific needs of the plan.
The simplest and least expensive approach to structured learning is outcome monitoring. This entails tracking the objectives of a plan to determine whether the expected outcomes are being realized. This type of monitoring supports trial and error learning: if one approach fails, try another.
A noteworthy variant of outcome monitoring involves decisions with embedded management thresholds or triggers (Cook et al. 2016). In these applications, monitoring is used as a feedback mechanism for actuating predetermined management decisions. This approach is commonly used for managing air quality and water quality/quantity. For example, water allocation rules may automatically change in the face of changing water supply (see Case Study 2). This approach permits management actions to adjust to changing conditions without having to wait for the next planning cycle.
Adaptive Management
Monitoring can also be applied as a structured learning tool to improve the predictive models that support decision making. This is referred to as adaptive management (Williams et al. 2009). Monitoring efforts are focused on the components of the model that have a large influence on the outcomes and are subject to high uncertainty. The intent is to fill gaps in knowledge and to validate the assumptions in the model, including implementation aspects. The overall aim is to improve the reliability of the predictive model, resulting in more informed decisions in the future.
Adaptive management is fundamentally a form of research. The hypotheses it seeks to test are the assumptions embedded in the predictive model. The study area is typically the planning area, though useful knowledge can also be gained from observations made elsewhere. For example, we might contrast observations made within the planning area, which we are perturbing through management actions, with observations made in natural areas.
Box 10.7. The Evolution of Adaptive Management
The adaptive management concept arose in the 1970s from frustrations resource managers had in applying models to real-world management problems (Walters and Hilborn 1978). Models were plagued by uncertainties that were inconvenient or costly for scientists to study because they involved processes that unfolded at large spatial and temporal scales. Adaptive management addressed this problem by using management actions as experimental treatments.
Adaptive management quickly gained adherents due to its inherent appeal. It was widely seen as a means to manage responsibly in the face of uncertainty. However, in practice, the adaptive management label was often applied indiscriminately to monitoring programs that did not feature structured learning based on predictive models. Furthermore, because adaptive management was initially conceived as a research tool, it proved difficult to integrate into the broader decision-making system, and this hampered its effectiveness (Walters 2007).
The adaptive management concept has evolved to address these shortcomings, and its role has been clarified. Today, it is seen as an integral component of the SDM cycle, rather than a stand-alone approach to research (Williams et al. 2009; Runge 2011).
Adaptive management initiatives can be differentiated into observational and experimental forms. Observational studies simply monitor what happens in response to the implementation of a preferred management alternative. In experimental studies, multiple management approaches are implemented in parallel as experimental treatments (Grantham et al. 2010). The observational and experimental forms of adaptive management are often referred to as “passive” and “active” forms, respectively (Williams 2011). However, these labels are not used consistently and are best avoided.
The appeal of the experimental approach is that it provides the fastest rate of knowledge gain. It also leverages the resources and management authority that are available to resource managers, enabling landscape-scale experiments that might otherwise be impossible to do. Experimentation epitomizes the concept of “learning by doing” that is central to adaptive management. Unfortunately, despite its appeal, there have been relatively few successful applications of experimental adaptive management (Walters 2007). In practice, it is difficult to secure the funding, staff, and institutional support needed for large-scale, long-term studies. Furthermore, it is often hard to obtain stakeholder support for experimentation in real-world settings.
An alternative to landscape-scale experiments is to conduct smaller-scale research studies focusing on specific processes. For example, forestry companies have long benefited from greenhouse experiments. Another option is to conduct pilot studies that involve just a portion of the planning area (Box 10.8).
Given the wide range of learning options available, the choice of which approach to use (if any) can be a difficult one. The decision should be based on a formal evaluation and comparison of the available options (Gregory et al. 2006). Detailed analysis is particularly appropriate when the management stakes are high and large sums of money are involved.
The main considerations are the costs, benefits, and likelihood of success of the various monitoring and research options (Gregory et al. 2006). Costs are measured in terms of the time and financial resources needed to implement the programs. Benefits are measured in terms of the ability of the acquired knowledge to improve future decisions. The assessment of the likelihood of success considers the ability of a learning program to deliver what it promises (Williams et al. 2009). The feasibility of implementing the program and the ability to generate statistically meaningful results are important factors. Institutional factors also need to be considered, including the level of commitment, capacity for sustaining a long-term program, systems for appropriately managing the data, and mechanisms for applying the findings to future decisions.
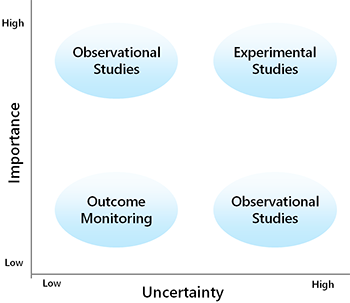
In addition to determining which learning approach will provide the best information for a given budget, there is the question of what the learning budget should be. This is a resource allocation issue that pits the benefits of learning against other management objectives (Gregory et al. 2006). As a general rule, learning should be emphasized when uncertainty about a decision is very high, particularly for issues of great importance (Fig. 10.10). There is little logic in implementing costly management actions when we are unsure of what actions to take. On the other hand, when the necessary management actions are abundantly clear, there is little justification for diverting funds to expensive research programs.
With all forms of monitoring, it is important to have a good information management system in place. Data should be consistently recorded, well organized, and easy to access. In addition, formal linkages should be in place to ensure that the collected data are incorporated into the next decision-making cycle. An effort should also be made to publish case study reports to facilitate learning in other areas.
Box 10.8. Learning Through Pilot Studies
Learning through passive monitoring is a slow process. Large-scale adaptive management experiments are much more effective but difficult to implement in practice. Pilot studies offer a middle road, providing a way to break complex problems into tractable components, and to test innovations at a small and manageable scale (Brunner and Clark 1997). These studies allow for flexible implementation in case of unexpected problems or opportunities. Indeed, an aim of all pilot studies is to devise a better program as experience is gained.
The small scale of pilot studies helps them maintain a low profile. This minimizes political visibility, and therefore vulnerability, until the results have been evaluated. If unsuccessful, a pilot study can be terminated more easily than a full-scale intervention because it is less likely to have acquired a large constituency willing to defend it. If successful, it can be expanded laterally and incorporated in the next decision cycle as a new and viable management option.